Understanding Data Annotation in Machine Learning
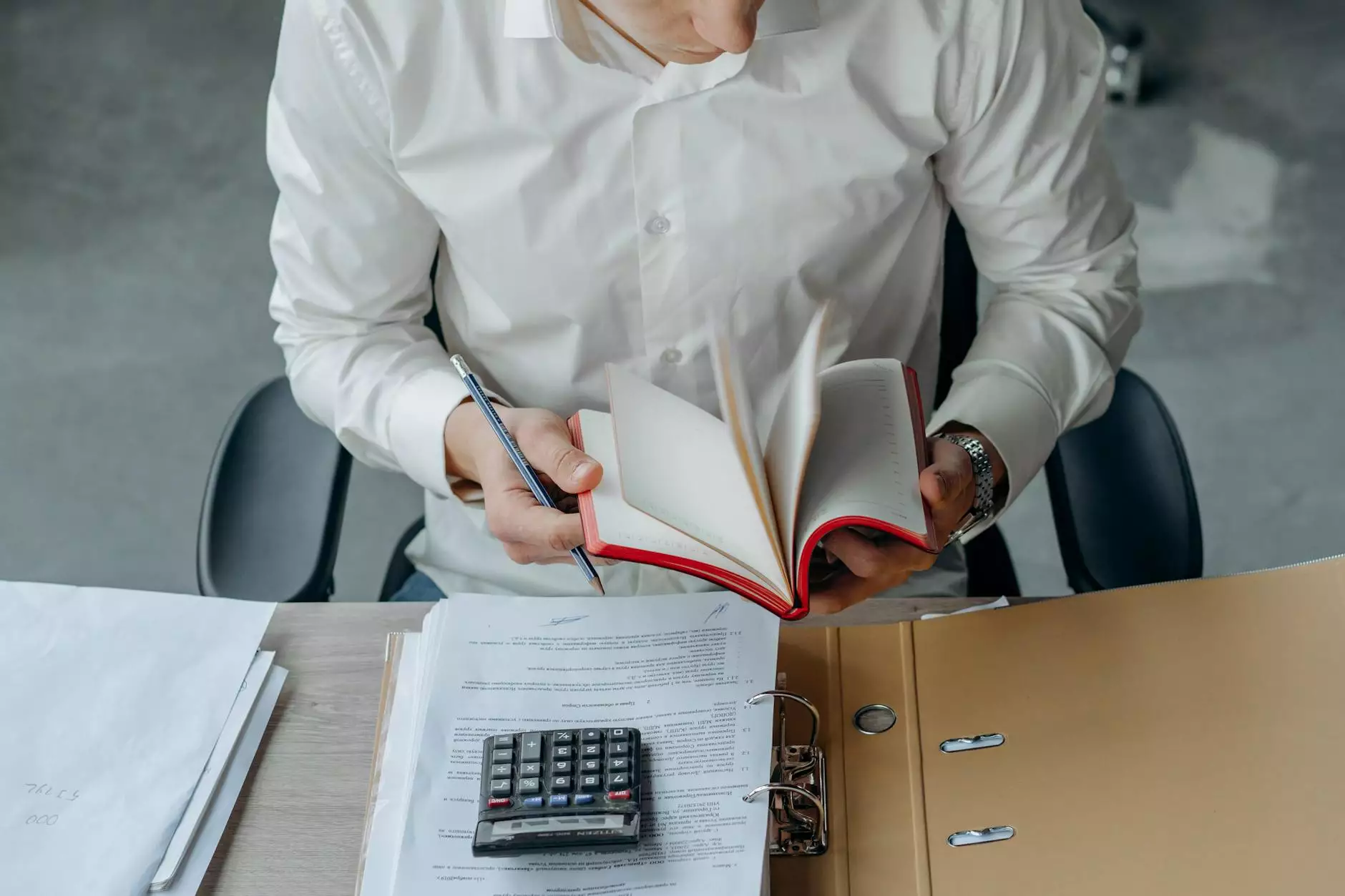
Data annotation plays a crucial role in the world of machine learning. As businesses and technology evolve, the demand for accurate, well-annotated data grows exponentially. In this article, we will dive deep into the significance of data annotation, its applications in various industries, and how companies, especially in the realms of home services like KeyMakr, benefit from it.
What is Data Annotation?
At its core, data annotation refers to the process of labeling or tagging data to provide context and meaning. This involves providing various types of labels to data such as images, text, audio, and video. In machine learning, annotated data is essential for training algorithms to recognize patterns and make predictions.
The Importance of Data Annotation in Machine Learning
The relevance of data annotation cannot be overstated. Here are some key reasons why effective annotation is vital:
- Accuracy: Properly annotated data ensures that machine learning models can make accurate predictions.
- Efficiency: Well-annotated datasets can speed up the training process, leading to quicker deployment of AI solutions.
- Quality of Output: The output of a machine learning system is only as good as the data it learns from.
Types of Data Annotation
There are several types of data annotation techniques, each serving different purposes. Here are some common methods:
- Image Annotation: This includes labeling images for object detection, segmentation, and classification. It helps in training computer vision applications.
- Text Annotation: This involves tagging parts of text for sentiment analysis, named entity recognition, and other NLP tasks.
- Audio Annotation: This is the process of identifying and tagging audio clips for applications such as speech recognition.
- Video Annotation: This includes labeling objects or actions in video sequences for applications in surveillance and autonomous vehicles.
Applications of Data Annotation in Business
Data annotation has transformed numerous sectors, including:
1. Healthcare
In the healthcare industry, annotated medical data can assist in diagnostic imaging and patient data analysis, enhancing decision-making and patient care.
2. Automotive
Autonomous driving technology heavily relies on annotated video data to train vehicles to detect and interpret their surroundings effectively.
3. E-commerce
Data annotation enhances product categorization and customer sentiment analysis, enabling businesses to provide personalized shopping experiences.
4. Security
In surveillance systems, annotated video data helps in refining pattern recognition algorithms, improving security protocols.
KeyMakr: Leveraging Data Annotation for Enhanced Home Services
KeyMakr, a leader in the home services industry, understands the importance of integrating advanced technologies like data annotation into its offerings. By utilizing machine learning algorithms that rely on well-annotated data, KeyMakr can:
- Improve Service Efficiency: Automation through machine learning can streamline service requests and responses, enhancing customer satisfaction.
- Enhance Security: Using video and image data annotation, KeyMakr can improve the safety protocols surrounding locksmith services.
- Personalize Customer Interaction: Analyzing customer data helps in providing tailored services, addressing clients' unique needs.
Best Practices for Data Annotation
For businesses to maximize the benefits of data annotation, certain best practices should be followed:
- Choosing the Right Tools: Utilize reliable data annotation tools that fit the specific needs of your business.
- Ensuring Quality Control: Implementing a validation process to ensure the accuracy and consistency of annotated data.
- Training Annotators: Providing comprehensive training to those involved in the annotation process enhances quality and efficiency.
- Regular Updates and Reviews: Continuously updating and refining the datasets as new data comes in maintains the relevance and accuracy of the training data.
The Future of Data Annotation in Machine Learning
As AI continues to evolve, the future of data annotation looks promising. Here are some trends that indicate where the industry is headed:
- Increased Use of Automation: Tools that automate part of the annotation process will become more common, although human oversight will remain critical.
- Integration with AI Tools: AI will play a larger role in suggesting annotations and streamlining processes.
- Enhanced Focus on Ethics: With growing concern over data privacy, ethical considerations in data annotation will become paramount.
Conclusion: Embrace Data Annotation for Business Growth
In today's data-driven environment, understanding data annotation in machine learning is essential for any business looking to thrive. Companies like KeyMakr demonstrate the potential benefits of leveraging this practice for improved service delivery, enhanced customer interaction, and ultimately, business success. By prioritizing data annotation, businesses can not only stay competitive but also lead their industries into the future.
If your enterprise is ready to harness the power of data annotation, it’s time to explore the right tools, strategies, and techniques to effectively implement this critical aspect of machine learning.
data annotation machine learning